New machine learning model for predicting infrared spectra of ions
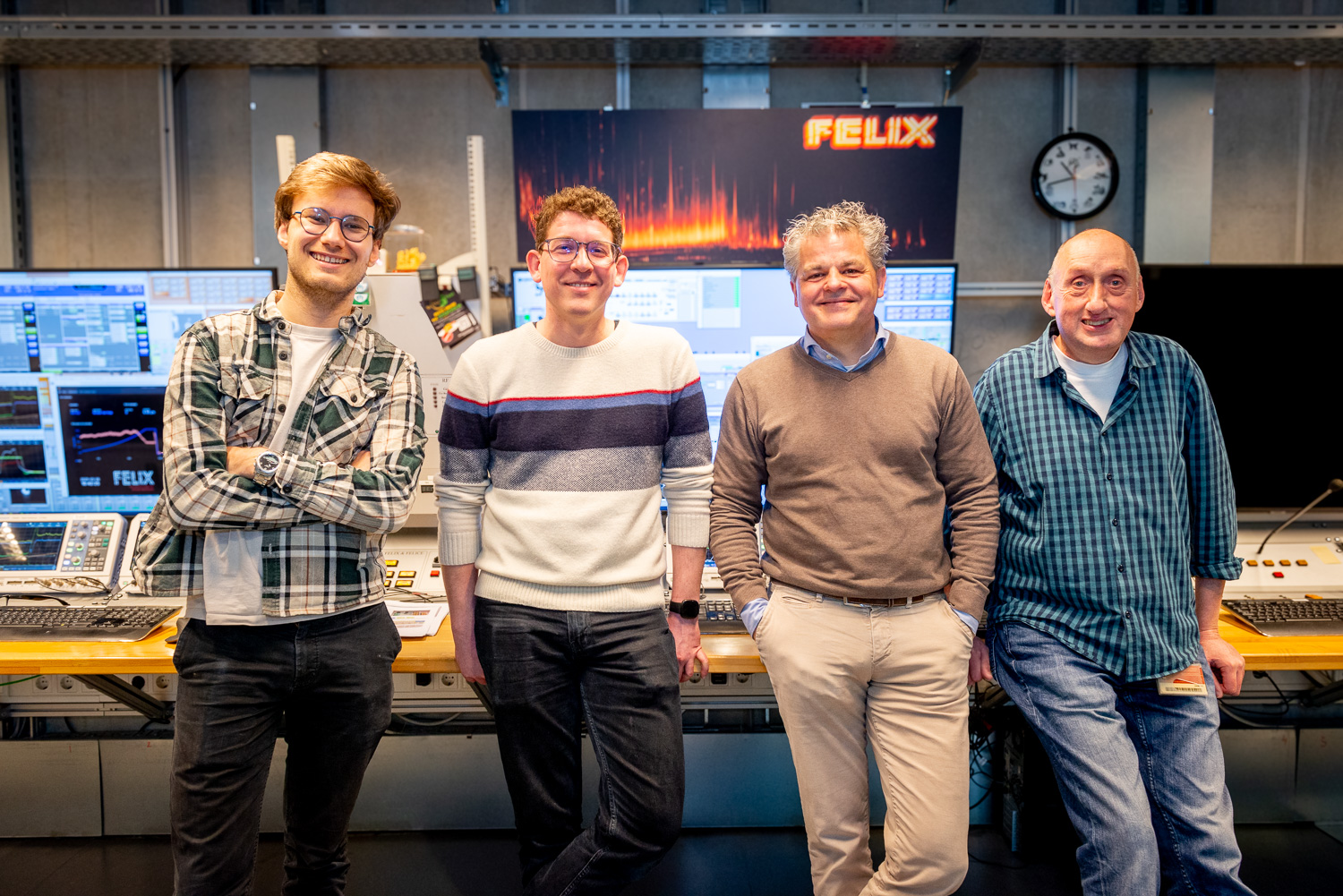
HFML-FELIX researchers Teun van Wieringen, Jonathan Martens, Jos Oomens and Giel Berden.
Researchers at HFML-FELIX and their collaborators at the University of Waterloo in Canada have built and demonstrated a novel machine learning model that is able to predict infrared spectra of ions much faster and with better accuracy than traditional models.
The broad aim of this research is to identify molecular unknowns. Think of, for instance, blood samples from a group of patients with a common disease or common set of symptoms. It is already known the patients in this group have molecules in their bodies that differ from healthy people, so called biomarkers. The laboratory tests at the hospital can measure the masses of these molecules, but they do not have the techniques to determine the molecular structures. This poses a problem for molecules that are not previously associated with a particular disease or are previously unknown.
‘If you want to use these molecules as biomarkers for a specific disease’, Jonathan Martens of HFML-FELIX explains, ‘you will have to know their structures as well. There might be several molecules with the same mass, but they can have very different functions in the body. And that is where we come in. We use one of our infrared lasers – FELIX – to identify the structure of these unknown molecules.’
Much faster
Interpretation of infrared spectra of ions to determine molecular structures can be challenging and has traditionally relied on quantum chemical modelling. ‘That is why we use computer models; so we can predict the infrared spectrum of likely candidates and then see which one matches what we find in experiments.’
However, predicting the infrared spectrum or ‘fingerprint’ for candidate structures can be time consuming. Just one calculation can take from several hours up to several days and calculating the infrared spectra for all candidate molecules of an unknown substance can even take weeks. ‘A model like the one we have developed here, gives you a spectrum every few seconds. This enables you to explore a much wider chemical space to find your match and you can do this in a fraction of the time.’
Many applications
It is a promising model not only for clinical analysis in the health sector, but also for environmental analysis and forensics. However, this new model has been developed using a very limited training set. So follow up studies will be necessary to test what chemical data sets it does and does not work for. ‘We expect it to do very well on a variety of chemical data sets, but we will have to run more tests to know for sure.’
There already are other models that predict the infrared spectra of molecules. Large ones, trained on tens of thousands of molecules. That is a lot more than the 300 that can be found in this new model. So what makes it better? ‘In these large sets the molecules are almost entirely neutrals. The model we tested at HFML-FELIX looked at ions. The data set might be more limited, but the applicability to ions is extremely valuable. Mass spectrometry is one of the most commonly used techniques in modern analytical chemistry for the analysis of complex mixtures, and this technique works with ions, not neutral molecules. The model developed in this study allows us to predict the infrared spectra of unknown ions detected in mass spectrometry experiments and then to determine their molecular structures.’
More accurate
What they saw with this particular training and test set is that the new machine learning model outperforms quantum chemical methods by 21 percent in terms of accuracy. ‘It will probably not be representative for all chemical systems’, Martens says, ‘but over the limited test set we have used, it performs significantly better. And I fully expect that if we can expand the data set used for training then the model will become more versatile as well.’ This achievement in accuracy despite the scarcity of infrared spectra is in fact the real breakthrough here. ‘The transfer-learning approach used in training the model seems to have really worked very well.’
One of the more challenging aspects of this study was making sure the model wasn’t just predicting things that were very similar to what was in the training data. ‘We needed to know if it was “learning” and improving. Whether it could also predict molecular types that were similar, but not an exact match of a molecule in the training data. It turned out it could, so then we knew the model was doing what it was supposed to do.’
Future breakthroughs
Like Martens said before there will be follow up research to improve the model even further. If they succeed, they could be on to something even more exciting. Something that will change the research field all together. ‘It would be even more valuable if we could turn the whole process around. What if we don’t finish with a list of infrared spectra, but we start with that. The spectrum would be the input for the model and what comes out is the molecule. This is not easy, but it would be a massive breakthrough if we can make that work.’
Out of any given blood sample, we now are routinely able to understand only a few percent of everything that is in there. It can contain thousands of compounds that can tell you something about someone’s health, but most of those features come back as complete unknowns. If they could turn it around and put the infrared spectra of the unknowns in a model that then predicts the molecule, this would be revolutionary as it would massively speed up the entire process. It could, for example, enable very precise personalized therapies and monitoring and we would understand the body and its workings a lot better than we already do.
For now, researchers studying unknown molecules have gained a very useful new model that can save them a lot of time. And that is something that is valuable, whether you are trying to determine what disease you are dealing with, testing the quality of water, or trying to identify novel psychoactive substances.
You can find the paper here: A Machine-Learned “Chemical Intuition” to Overcome Spectroscopic Data Scarcity
Research contact: Jonathan Martens